Learning Generalizable Policies for Assembly Sequence Planning
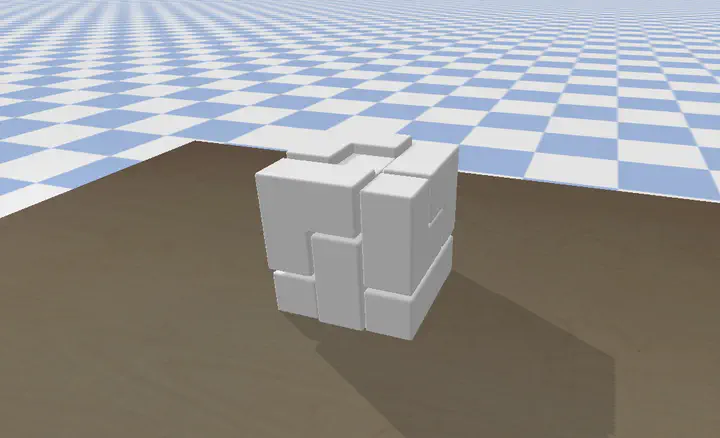
• Formulate the robot assembly sequencing as a combinatorial optimization problem over graph and solve it with a generalizable graph-based reinforcement learning framework
• Build a physics simulation environment to generate feasibility constraints, i.e., interference matrices and stability matrices, of the assembly objects with PyBullet
• Implement the graph neural networks to encode the state of the environment and build the reinforcement learning algorithm to learn a task and motion planner for the assembly sequence planning